Obama, Trump, and Biden walk into a bar and talk about baking gingerbread cookies. This scenario has likely never happened in real life, but on TikTok, you can find an audio recording of this conversation happening, down to the correct voices and all. It’s pretty obvious from the context that the recording is fake, and most people who encounter it will probably find it funny, regardless of what side of the political spectrum they’re on. But the recording itself begs the question: What if we didn’t have that context to know that the recording was fake? What if the three presidents had been discussing something other than baking gingerbread cookies? Given that people now have the technology to create videos of anyone doing anything, how can we tell what is real and what is not?
These types of social media videos are examples of deepfakes—AI–generated images or recordings of people doing and saying things that they never actually did. Deepfake technology itself has been around for over 20 years, and it was first used by movie studios to dub over clips of actors speaking and artificially alter their lip–movements to fit new audio. In 2016, the Technical University of Munich’s project Face2Face expanded the capabilities of deepfake technology, by allowing users to translate their own mouth movements onto the faces of famous people. Shortly afterward, researchers at the University of Washington were able to train a neural network on hours of Obama’s speeches and produce a realistic but fake video of him talking.
Although deepfake technology was already in development before the advent of social media, deepfakes have been gaining more public awareness and notoriety since 2017, when a Reddit user began creating and posting deepfake pornography of mainly female celebrities. Since then, deepfakes have been used in a wide range of contexts—from humorous to political to exploitive—prompting efforts by the United States Congress and social media platforms to navigate this newly–emerging digital landscape that challenges our most fundamental assumptions of what is real and what is not.
Avery Hong (N ‘27) who has been using social media since seventh grade, voiced his concerns about false AI–generated content online. He points to an example of a deepfake video of Taylor Swift singing a rap song, something that he found funny because he could tell that it was fake, while simultaneously concerned by the realism and accuracy of the video.
“As technology advances more, I feel some stuff can be real, some stuff can be fake, and I [wouldn’t be able] to tell,” he says. “That’s the most concerning part for me … you can deepfake anything and people can believe it.”
Robert Ghrist is the Penn Engineering associate dean for undergraduate education and specializes in mathematics research with a focus on networks and data. Generative AI, he says, is meant to make things up. A popular misconception is that generative AI is meant to produce a certain correct result, similar to a search engine, but Ghrist points out that [the program] merely seeks to produce content that it believes—in the anthropological sense of the word—will make users happy.
Deepfakes, a product of generative AI, are created by linking two neural networks together to create a Generative Adversarial Network (GAN). The first neural network in the GAN, the “generator,” creates pictures of people. The second neural network, the “discriminator,” determines whether a picture of a person is real or fake. Within the GAN, the generator creates a picture of a person, and the discriminator guesses whether it is real or not. If the generator produces a fake image, but the discriminator does not recognize it as fake, then the discriminator must evolve to do a better job at telling what is real and what is not.
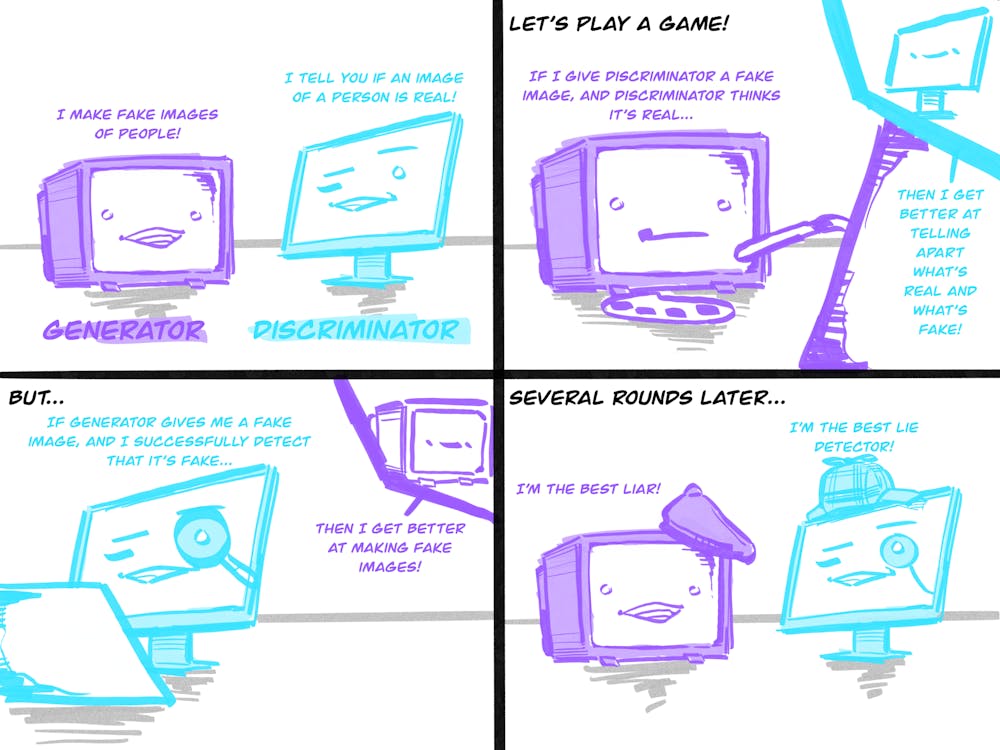
But if the generator produces a fake image, and the discriminator recognizes that it’s fake, then the generator evolves and gets better at producing fake images. When you force the generator and discriminator to compete against each other many times, you eventually reach an “equilibrium where the discriminator is as good as can be at telling the difference between real and fake, but the generator is as good as can be at generating things that you can’t tell whether it’s real or not,” Ghrist explains.
Ghrist maintains that it’s important to understand how the GAN works in the context of trying to figure out how to control generative AI. “Everyone wants to build the perfect detector for identifying fake news,” Ghrist says. “As soon as you build a really good fake news detector, all you have to do is hook it up to a generator, build a GAN, then voila, you have the perfect propaganda machine.” The way Ghrist sees it, people can either give up and stop using AI—something that we are far past the point of—or evolve as human bodies do when new viruses appear. “Unfortunately, the way evolution goes, there’s a lot of damage until we get an evolved immune system,” he acknowledges.
Cary Coglianese, a professor of law at Penn and the director of the Penn Program on Regulation, specializes in regulatory law and has been working on issues related to government use and regulation of AI. Machine learning, he says, encompasses a broad array of strategies, algorithms, and technologies that can produce remarkable results, such as identifying flight passengers with COVID–19 and spotting signs of cancer in radiographs. Given that computers can process thousands of more variables than humans can at once, AI provides users with the means of achieving things that would otherwise be difficult, if not impossible, for humans, including “cancer detection … and sowing discord in society.”
With the wide range of helpful and harmful applications of AI, it is difficult to know how to avoid restricting the benefits of AI while still keeping people safe from its risks. Coglianese compares AI regulation to putting a leash on a dog, allowing it to wander while still making sure that it won’t get itself into trouble. “I think that we need to find regulatory tools that allow some degree of innovation and flexibility while also putting the incentives and the responsibilities on firms to identify the problems with their tools, as well as provide adequate human oversight in their development, application, and ongoing use,” he explains.
Coglianese believes that this can be achieved through a type of management–based regulation. Due to the variations in AI use, there is no one–size–fits–all solution to fixing AI’s issues. Rather, a management–based approach puts the onus on companies to create internal procedures to assess, minimize, and monitor the risks of their AI tools. This management–based approach is already being used in food manufacturing and cybersecurity regulations; while it is not foolproof, Coglianese believes that it is the “best strategy that we have available.”
Coglianese acknowledges that, while there are legal actions people can take if they have been harmed through a deepfake, it can be difficult to identify who first created the deepfake once it has been disseminated on social media. Still, he remains hopeful that the public will learn how to be more discerning about their sources of information. “I think many people are aware that deepfakes exist … hopefully, that’s a non–legal, but sociological counterbalance to the deepfake problem,” he says.
When it comes to his concerns about generative AI, Ghrist discusses the possibility of users forming parasocial relationships with machines, as is conjecturally happening in the case of the chatbot Replika. Having seen the effects that social media has on teenagers, Ghrist says that it can be especially dangerous when young people put themselves in emotionally vulnerable positions with machines, and treat things that are not real as if they are. And while manually photoshopping an image to create a deepfake requires skill and expertise, generative AI tools are becoming increasingly easy to access, allowing “anybody to conjure anything into existence.”
The increasing prevalence of deepfake content has “changed everything” about the way that Avery interacts with information online. He is very skeptical about everything that he sees on Instagram these days; a recent example of misinformation he encountered was when fabricated documents showing Stephen Hawking being present on Jeffrey Epstein’s island were circulating around Twitter. Avery had to do external reading on CNN and The Guardian before he could find evidence that the information was false, an additional step of confirmation that most social media users do not take.
“I feel that people on the internet are just believing things that they see online too much … and they’ll see a deepfake and believe it,” he says in response to the incident. In this case, Avery points out how being able to convincingly fabricate information about people could increase wrongful blacklistings and cancelations, ruining careers and lives.
The impact of AI–fueled misinformation extends beyond just social media apps. Coglianese notes that one way regulations are created is by having an agency post the proposed rule online before it goes into effect, and people will be able to leave public comments on it for the agency to review and determine if any changes to the rule need to be made. “There’s a real concern about fake comments coming into the regulatory process in general through bot–created comments,” he explains.
Additionally, Coglianese voices a greater concern that deepfakes could be used to incite actions from people that are not based on facts, potentially creating a “tremendous amount of havoc.”
“We can see how people can be mobilized to action by seeing an individual putting themselves on fire or a police officer murdering a civilian,” he says. “Images move people, and if we are in a world in which images can be imagined and portrayed as being real when they’re not, and people believe them, that can create a lot of mischief.”
Ghrist is surprised that he has not encountered more deepfakes associated with political campaigns yet, and Coglianese illustrates a scenario in which a deepfake that “convinces 80,000 people out of 80 million voters to go one way could tip the balance at a presidential election,” leading to “huge consequences even if only a small number of people are affected by a deepfake.”
With all of the potentially disruptive consequences of deepfakes, there is the question of where these rapidly–developing technologies will lead us. OpenAI just announced their new model, Sora, that will allow users to generate videos from text prompts, eliciting concerns that users will be able to use it to produce deepfake videos more easily than ever before. While there have been recent calls for putting a six–month pause on generative AI technology, Coglianese believes that the internet is ultimately past that point. Still, he is hopeful that, through regulation, lawmakers will be able to “maximize [AI’s] benefits while minimizing the risks and harms.”
Avery would like to see a feature on social media platforms that displays whether a piece of created information is human or AI–generated. Ghrist points to a new feature on X, formerly Twitter, that allows users to crowdsource feedback on whether a post is true or not, and also requires them to provide citations for sources that are verified for trustworthiness. While the crowdsourcing feature is not a perfect solution, it is a much better approach than others that solely rely on the platform itself. Increased cryptography in cameras would be a viable possible solution, where any picture taken from a phone can be verified as being a real picture through its metadata. In the meantime, since deepfakes are often used to impersonate family and friends in scams, Ghrist recommends having a safe phrase among family members that is not written down anywhere and can be used to identify the identity of the person sending a message.
As the story of generative AI continues to unfold, each platform can independently experiment with solutions and allow them to evolve in parallel, enabling tech companies to determine which strategies work best. Ghrist predicts that the separation of social media platforms will be a valuable asset in teaching online communities how to respond to deepfakes.
With all of the public attention that AI deepfakes have been garnering, Coglianese acknowledges that lawmakers' perceptions about AI misuse could influence their views on AI as a whole. Similar to how environmental regulation is often affected by lawmakers’ individual beliefs about the environment, Coglianese points out that the predispositions of lawmakers against AI could affect the shape of AI legislation and politics.
With regards to the public sphere, Ghrist agrees that AI misuse on social media will likely elicit negative responses against generative AI as a whole, citing previous instances of panic regarding video games and rock and roll as examples. While he is “absolutely worried” that the prevalence of deepfakes may erode popular trust in AI, he also believes that, just like video games and rock and roll, public perceptions will eventually come around and “recognize that there’s a lot of good” in AI alongside the “potential for bad.”
“The general principle that affects how I order my life, how I do all kinds of things, including my job, is that I believe that adults should be as free as possible within the limits of society,” Ghrist says. There is a shared responsibility not to hurt others and to protect those who are not adults yet. But, for college students and older, Ghrist believes that they are capable of making their own choices about how to engage with technology and live their lives, subject to laws.
“Given the finite amount of resources that we have with respect to education and control, I think the best use of those resources is to teach people, especially young people, how to be wise, how to be responsible, and how to live in society,” Ghrist states. “You teach those core principles, and then no matter what tech gets developed, you’ve got the foundation and the basis for being able to handle it.”
A self–described “geek when it comes to generative AI,” Ghrist is earnest about AI’s potential, pointing out capabilities like automating “drudge–type” work, like writing corporate memos or saving human lives by assisting with drug discoveries. He recalls his job as an early college student where he used to spend hours feeding magnetic tapes into a bank’s mainframe computer. Now, he owns a flash drive that does that for him, a solution that’s a “billion times better and costs about 15 bucks.”
“I am envious of you and your young readers because you’re going to live in an exciting, exciting world,” Ghrist says. “My excitement is not without some trepidation for the adaptation that we’re going to have to do. But wow, I think that the things we’re seeing in generative AI is invention–of–the–printing–press–size innovation, and the world is going to change.”